How to choose the right server with the right CPU for your neural networks
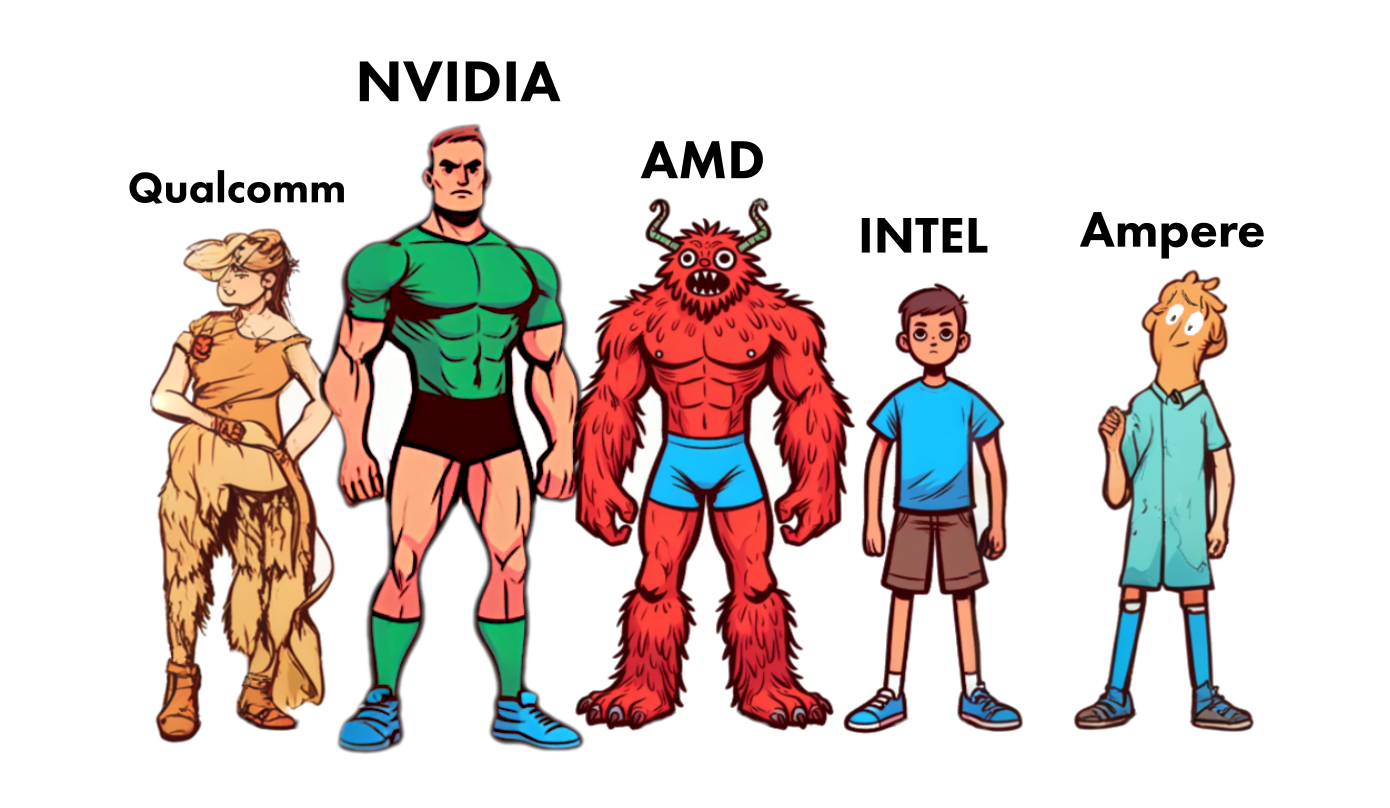
With the development of generative artificial intelligence (AI) and the expansion of its applications, the creation of servers with artificial intelligence has become critical for various sectors – from the automotive industry to medicine, as well as for educational and government institutions.
This article talks about the most important components that influence the choice of an artificial intelligence server – the central processing unit (CPU) and the graphics processing unit (GPU). Selecting the right processors and graphics cards will allow you to run a supercomputing platform and significantly speed up artificial intelligence computing on a dedicated or virtual server (VPS).
Rent dedicated and virtual GPU servers with professional graphics cards NVIDIA RTX A5000 / A4000 and Tesla A100 / H100 80Gb, as well as RTX4090 gaming cards in reliable TIER III class data centers in Moscow, the Netherlands and Iceland. We accept payment for HOSTKEY services in Europe in rubles to the account of a Russian company. Payment using bank cards, including the MIR card, bank transfer and electronic money. Server rental with hourly payment.
How to choose the right processor for your AI server?
The processor is the main “computer” that receives commands from the user and executes “command loops” that will produce the desired results. So a big part of what makes an AI server so powerful is the processor that sits at its heart.
You might be expecting to see a comparison between AMD and Intel processors. Yes, these two industry leaders are at the forefront of processor manufacturing, and Intel's fifth-generation Intel® Xeon® processor line (sixth generation has already been announced) and AMD's EPYC™ 8004/9004 line represent the pinnacle of x86-based CISC processor development .
If you are looking for excellent performance combined with a mature and proven ecosystem, then choosing the top products from these manufacturers will be the right decision. If your budget is limited, you may want to consider older versions of Intel® Xeon® and AMD EPYC™ processors.
Even older desktop processors from AMD or Nvidia are a good choice for getting started with AI if your workload doesn't require a lot of cores and limited multi-threading capabilities. In practice, for language models, the choice between CPU types will matter less than the choice between a graphics accelerator or the amount of RAM installed in the server.
Although some models (for example, 8x7B from Mixtral) can show results on a processor comparable to the computing power of tensor cores of video cards, they also require two to three times more RAM than in a CPU + GPU combination. For example, a model that will run on 16 GB of RAM and 24 GB of GPU video memory, when running only on the central processor, may require up to 64 GB of RAM.
There are other options besides AMD and Intel. These may include solutions from ARM architecture, such as NVIDIA Grace™, which combines ARM cores with proprietary NVIDIA or Ampere Altra™ features.
How to choose the right graphics processing unit (GPU) for your AI server?
The Graphics Processing Unit (GPU) plays an important role in running an AI server. It serves as an accelerator that helps the central processing unit (CPU) process requests to neural networks much faster and more efficiently. The GPU can break a task into smaller segments and process them simultaneously using parallel computing or specialized cores. The same NVIDIA Tensor Cores deliver orders of magnitude faster 8-bit floating-point (FP8) performance in the Transformer Engine, Tensor Float 32 (TF32), and FP16, and excel in high-performance computing (HPC).
This is especially noticeable not during inference (the operation of the neural network), but during its training, since, for example, for models with FP32 this process can take several weeks or even months.
To narrow your search, you need to find the answer to the following questions:
Will the nature of my AI workload change over time?
Most modern GPUs are designed to perform very specific tasks. Their chip architecture may be suitable for certain areas of AI development or application, and new hardware and software solutions may render the previous generation of GPUs uncompetitive in the next two to three years.
Will you be primarily involved in AI training or inference (exploitation)?
These two processes underlie all modern iterations of memory-constrained AI.
During training, an AI model consumes a large amount of big data with billions or even trillions of parameters. It adjusts the “weights” of its algorithms until it can consistently generate the correct result.
During inference, AI relies on its learning “memory” to respond to new inputs in the real world. Both of these processes require significant computing resources, so GPU cards and expansion modules are installed to speed up the work.
For artificial intelligence training, GPUs are equipped with specialized cores and mechanisms that can optimize this process.
For example, the NVIDIA H100 with 8 GPU cores is capable of delivering over 32 petaflops of deep learning performance in FP8. Each H100 contains fourth-generation Tensor Cores using the new FP8 data type, as well as a Transformer Engine to optimize model training. NVIDIA recently introduced the next generation of its B200 GPUs, which will be even more powerful.
A good alternative to AMD solutions would be the AMD Instinct™ MI300X. Its feature is huge memory and high data throughput, which is important for the inference mode of generative AI, such as large language models (LLM). AMD claims that its GPUs are 30% more efficient than NVIDIA solutions, although they lose out on software.
If you're willing to sacrifice a little performance to meet budget constraints, or if the data set you're training your AI on isn't that large, it's worth taking a look at other offerings from AMD and NVIDIA. For inference modes or when there is no need for uninterrupted operation under full load 24/7, “consumer” solutions based on Nvidia RTX 4090 or even RTX 3090 are suitable for training.
If you're looking for stability in long-term computation for training models, you might consider the Nvidia RTX A4000 or A5000 graphics cards. While the H100 on PCIe may be a more powerful solution (60-80% depending on the application), the RTX A5000 is more affordable and suitable for some tasks (for example, working with 8x7B models).
Among more exotic solutions for inference, you can pay attention to AMD Alveo™ V70, NVIDIA A2/L4 Tensor Core, Qualcomm® Cloud AI 100 cards. In the near future, AMD and NVIDIA are preparing to supplant Intel with the Gaudi 3 GPU in the AI training market.
Based on the above and taking into account software optimization for HPC and AI, we can recommend servers with Intel Xeon and AMD Epyc processors and GPUs from NVIDIA. For AI inference, you can use GPUs from RTX A4000/A5000 to RTX 3090, and for training and running multimodal neural networks, you should budget for solutions from RTX 4090 to H100.
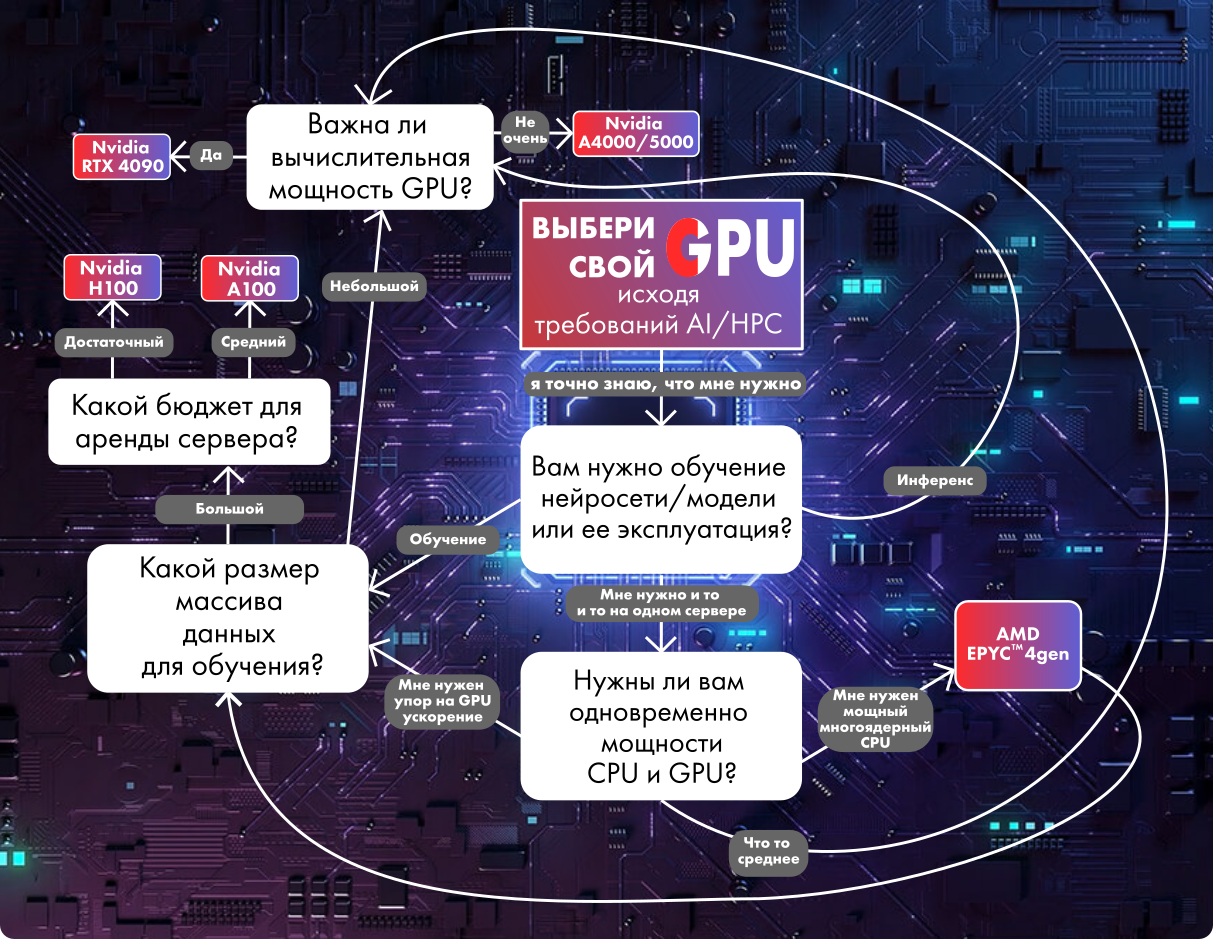
Rent dedicated and virtual GPU servers with professional graphics cards NVIDIA RTX A5000 / A4000 and Tesla A100 / H100 80Gb, as well as RTX4090 gaming cards in reliable TIER III class data centers in Moscow, the Netherlands and Iceland. We accept payment for HOSTKEY services in Europe in rubles to the account of a Russian company. Payment using bank cards, including the MIR card, bank transfer and electronic money. Server rental with hourly payment.