These strange, disturbing photos suggest AI is getting smarter

AI learns to generate images from signatures and begins to better understand our world
Of all the artificial intelligence models, it was OpenAI’s GPT-3 that captured the public’s imagination the most. She, without much prompting, can spew poems, short stories and songs, making you think that these are the work of a person. But eloquence is just a gimmick and should not be confused with real intelligence.
However, the researchers believe that the same techniques used to create GPT-3 hide a secret to create more advanced AI… GPT-3 was trained on a huge amount of text information. What if the same techniques were trained simultaneously on text and images?
A new study called AI2 from the Paul Allen Institute for Artificial Intelligence has taken this idea to the next level. Researchers have created a special, visual-linguistic model. It works with text and images and can generate the latter from signatures. The images look disturbing and strange, not at all like the hyperrealistic deepfakes created by generative adversarial networks (GANs). But they can show a new direction for more practical intelligence, and perhaps make robots smarter.
Fill in the gap
The GPT-3 belongs to a group of models known as “transformers”. They first gained popularity thanks to the success of BERT, Google’s algorithm. Before BERT, language models were pretty bad. Their predictive abilities were enough for autocomplete, but not for composing long sentences, where grammar rules and common sense are observed.
BERT changed the situation with the introduction of a new technique called “Masking” (approx. – original name masking). It implies that different words are hiding in the sentence, and the model should fill in the gap. Examples:
- The woman went to ___ to practice.
- They bought ___ bread to make sandwiches.
The idea is that if you force the model to do these exercises, often a million times, it will begin to detect patterns in how words are assembled into sentences and sentences are assembled into paragraphs. As a result, the algorithm generates and interprets the text better, coming closer to understanding the meaning of the language. (Google is now using BERT to deliver more relevant search results.) After masking proved to be extremely effective, researchers tried to apply it to visual language models by hiding words in signatures. In this way:
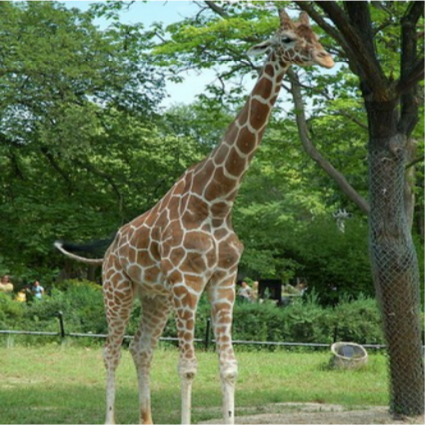
____ is on the ground next to a tree. Source: AI2
This time, the model could look at the surrounding words. and image content to fill the gap. After a million repetitions, she learned to detect not only the patterns of words, but also the connection of words with the elements of each image.
As a result, models can link textual relationships to visual examples of how babies make connections between learned words and things they see. Models can take the photo below and put together a meaningful caption, such as “Women play field hockey.” Or they might answer a question like “What color is the ball” by associating the word “ball” with a circular object in a photograph.
A visual-language model can form a meaningful caption for this photograph: “Women playing field hockey.” Source: JOHN TORCASIO / UNSPLASH
Better to see a picture once
The researchers wanted to know if these models develop a conceptual understanding of the visual world. A child who has learned a word for an object can not only name it, but also draw the object according to the hint, even if the object itself is absent. So the AI2 project team suggested that the models do the same: generate images from captions. All models produced meaningless pixel trash.
Is it a bird? This is a plane? No, this is gibberish, generated by artificial intelligence. Source: AI2
It makes sense: converting text to an image is more difficult than doing the opposite. “The signature doesn’t define everything in the picture,” says Ani Kembhavi, AI2’s computer vision team leader. Thus, the model must draw on a large amount of knowledge about our world to add missing details.
For example, if a model is asked to draw a “giraffe walking along a road,” then she needs to conclude that the road will be gray rather than bright pink, and will pass next to a field rather than the sea. Although all this information is not explicit.
So Kembhavi and his colleagues Jemin Cho, Jiasen Lu, and Hannane Hajishirzi decided to see if they could teach the model all this hidden visual knowledge by tweaking the approach to masking. Instead of training the algorithm to simply predict the “masked” words in the captions of the corresponding photographs, they also trained it to predict the “masked” pixels in the photos based on the corresponding captions.
The final images created by the model are not entirely realistic. But it is not important. They contain the correct high-level visual concepts. AI behaves like a child drawing a dash stick to represent a person. (You can test the model yourself here).
Sample images generated by the AI2 model from captions. Source: AI2
The ability of visual language models to generate this kind of image represents an important step forward in artificial intelligence research. This suggests that the model is actually capable of a certain level of abstraction – a fundamental skill for understanding the world.
In the long term, the skill can have important implications for robotics. The better the robot understands the environment and uses language to communicate, the more complex tasks it will be able to perform. In the short term, Hajishirzi notes, visualization will help researchers better understand what exactly the model is learning, which is now working like a black box.
In the future, the team plans to experiment more, improve the quality of image generation and expand the visual and vocabulary of the model: to include more topics, objects and adjectives.
“The creation of the images was really the missing piece of the puzzle,” says Lu. “By adding it, we can teach the model to better understand our world.”