The father of artificial intelligence Jeff Hinton: “Deep learning can do anything”
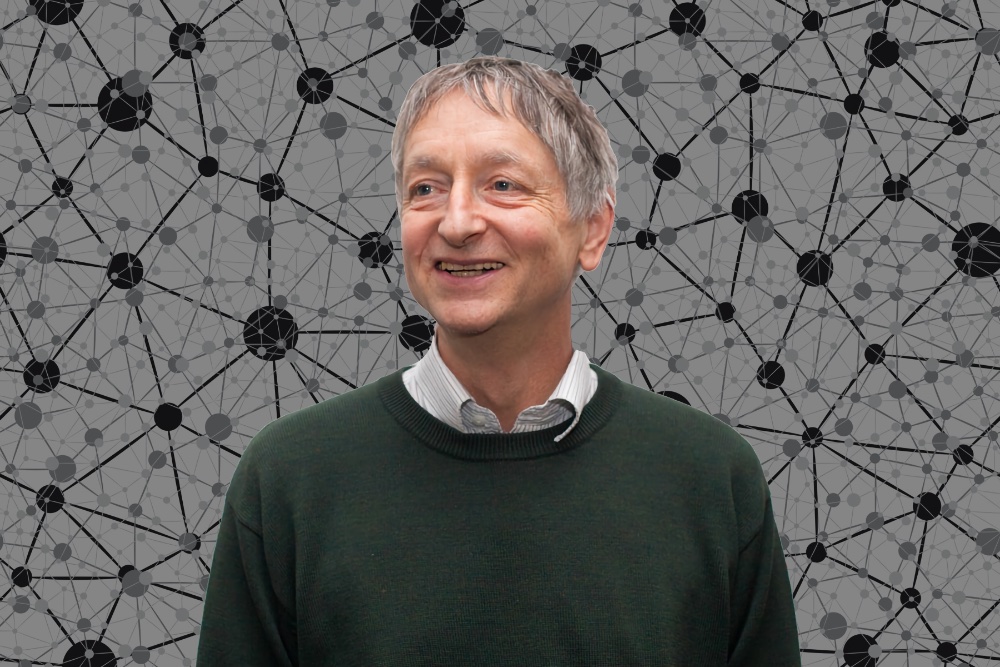
The modern artificial intelligence revolution began with a discreet research competition. This happened in 2012, the third year of the ImageNet competition. The challenge for the teams was to build a solution that would recognize a thousand images, from animals and people to landscapes.
In the first two years, the best teams did not achieve more than 75% accuracy. In the third year, a team of researchers – a professor and his students – suddenly broke through that ceiling. They won the competition by a staggering 10.8%. The professor’s name was Jeffrey Hinton, and his method was deep learning.
Hinton has worked with deep learning since the 1980s, but efficiency has been limited by lack of data and computational power. His steadfast faith in the method ultimately paid huge dividends. In the fourth year of the competition, nearly every team applied deep learning, achieving miraculous gains in accuracy. Soon, deep learning began to be applied in various industries, and not only in image recognition tasks.
Last year, Hinton, along with artificial intelligence pioneers Jan Lecun and Joshua Bengio, were awarded the Turing Prize for Foundational Contributions to this field of science.
You think deep learning will be enough to replicate all of human intelligence. What makes you so confident?
I do believe deep learning can do everything, but I think there should be quite a few conceptual breakthroughs. For example, in 2017 Ashish Vaswani and his colleagues introduced transformers that output really good vectors representing the meanings of words. It was a conceptual breakthrough. It is now used in almost all of the very best natural language processing. We will need a bunch of such breakthroughs.
And if we have such breakthroughs, will we bring artificial intelligence closer to human intelligence using deep learning?
Yes. Especially the breakthroughs related to how you get large vectors of neural activity to implement things like thinking. But we also need a huge scale. The human brain has about one hundred trillion parameters or synapses. The really big model we now call the GPT-3 with 175 billion parameters. This is a thousand times smaller than the brain. GPT-3 can now generate pretty believable text, but it’s still tiny compared to the brain.
When you talk about scale, do you mean large neural networks, data, or both?
Both. There is a mismatch between what happens in computer science and what happens in humans. People have a huge number of parameters compared to the amount of data they receive. Neural networks do surprisingly well with much less data and more parameters, but humans are even better.
Many AI specialists believe that AI sanity is an ability that needs to be pursued further. Do you agree?
I agree that this is one of the most important things. I also think movement control is very important, and deep learning networks are getting good at it today. In particular, some recent work by Google has shown that it is possible to combine fine motor skills and speech in such a way that the system can open a desk drawer, remove a block, and tell in natural language what it has done.
For things like GPT-3, which generates great texts, it’s clear that it has to understand a lot in order to generate text, but it’s not really clear how much it understands. But when something opens a drawer, takes out a block and says, “I just opened a drawer and took out a block,” it’s hard to say that it doesn’t understand what it is doing.
AI specialists have always looked at the human brain as an inexhaustible source of inspiration, and different approaches to AI have stemmed from different theories of cognitive science. Do you think the brain is really constructing an idea of the world in order to understand it, or is it just a useful way of thinking?
In cognitive science, there has long been a debate between the two schools of thought. The leader of the first school, Stephen Kosslin, believed that when the brain operates with visual images, we are talking about pixels and their movements. The second school was more in line with traditional AI. Its adherents said: “No, no, this is nonsense. These are hierarchical, structural descriptions. The mind has a certain symbolic structure, we control this very structure. “
I think both schools made the same mistake. Kosslin thought we were manipulating pixels because external images are made up of pixels and pixels are the representation we understand. The second school thought that because we manipulate symbolic representation and represent things through symbols, it is symbolic representation that we understand. I think these errors are equivalent. Inside the brain are large vectors of neural activity.
There are people who still believe that symbolic representation is one approach to AI.
Quite right. I have good friends, like Hector Levesque, who really believes in the symbolic approach and has done a great job in this sense. I disagree with him, but the symbolic approach is a perfectly reasonable thing to try. However, I think that in the end we realize that symbols just exist in the outside world, and we perform internal operations on large vectors.
What view of AI do you consider the most opposite to others?
Well, my problem is that I had these opposing views, and after five years they went mainstream. Most of my opposing views from the 1980s are now widely accepted. Nowadays it is quite difficult to find people who disagree with them. So yes, my opposing views have been undermined in some way.
Who knows, maybe your views and methods of working with AI will also be in the underground, and in a few years will become the industry standard. The main thing is not to stop in your progress. And we will be happy to help you with this by giving a special promotional code. HABR, which will add 10% to the banner discount.
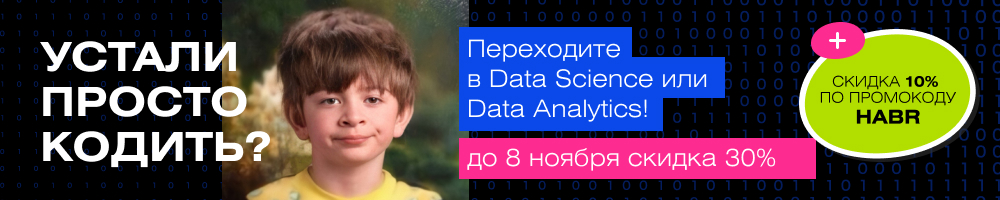
- Advanced Course “Machine Learning Pro + Deep Learning”
- Course “Mathematics and Machine Learning for Data Science”
- Machine Learning Course
- Data Science profession training
- Data Analyst training
- Data Analytics Online Bootcamp
- JavaScript course
- Profession Web developer
- Java developer profession
- Python for Web Development Course
- SQL for data analysis
- C ++ developer
- Data Analytics Course
- DevOps course
- The profession of iOS developer from scratch
- Profession Android developer from scratch
Recommended articles
- How to Become a Data Scientist Without Online Courses
- 450 free courses from the Ivy League
- How to learn Machine Learning 5 days a week for 9 months in a row
- How much data analyst earns: overview of salaries and vacancies in Russia and abroad in 2020
- Machine Learning and Computer Vision in the Mining Industry