Online speech synthesis for people with neurological disorders
Many people lose the ability to maintain their independence, lead an active social life and simply interact with the environment due to serious neurological diseases.
Diseases and conditions such as ALS, stroke, cerebral palsy, multiple sclerosis, Parkinson's disease, consequences of traumatic brain injury, dystoniacan lead to partial or complete loss of the ability to move independently, speak and perform daily tasks. Restoring these functions is very difficult, and in some cases completely impossible.
Many researchers are working on this problem, fighting to improve the standard of living for such patients.
In this article I will talk about testing the possibility of using the technology implantable brain-computer interface for online speech synthesis using brain activity recorded by intracranial electrodes to pave the way for new communication capabilities for people who have lost the ability to speak.
Happy reading!
Introduction to the Study
Various neurological disorders, including amyotrophic lateral sclerosis (ALS)can severely impact speech production and other goal-directed movements while preserving cognitive abilities.
Amyotrophic lateral sclerosis (ALS) is an incurable disease that destroys the cells that transmit motor nerve impulses. As a result, the muscles stop receiving commands from the brain, gradually weaken and atrophy.
Such diseases can lead to a variety of communication disorders, including locked-in syndrome, in which patients can only answer yes/no questions or choose from sequentially presented options using blinks, eye movements, or other residual movements.
In connection with this problem, research has begun based on the use of implantable brain-computer interface (BCI) for people with similar disorders.
An implantable brain-computer interface (BCI) is a technology that is implanted inside the body and allows a person's brain to be directly connected to a computer system. The purpose of such an interface is to translate the brain's neural activity into commands that can control external devices or software, bypassing traditional nervous system pathways such as muscle movements that may be impaired by injury or disease. This opens up new opportunities for people with severe physical disabilities to communicate, control and interact with the world around them.
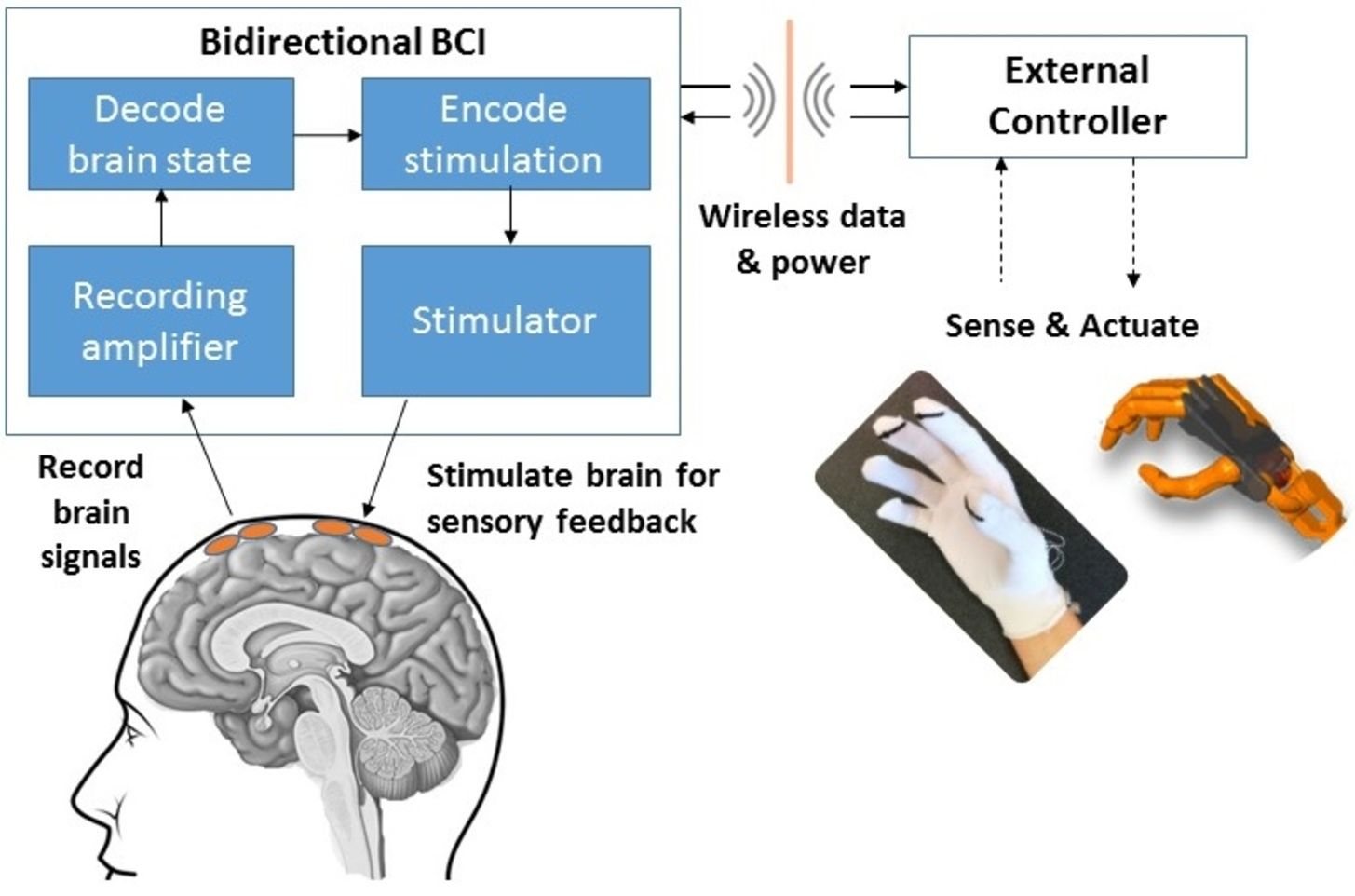
The principle of operation of the implantable brain-computer interface (BCI) is as follows:
Data collection: Implanted electrodes placed in specific areas of the brain collect electrical signals (ECoG signals) that reflect the neural activity of the brain. These signals can be received in real time and represent the user's specific thought processes or motor intentions.
Analysis and decoding: The collected data is transferred to an external device, where special software analyzes and decodes the signals. This involves recognizing patterns that correspond to certain mental commands or tasks.
Translation into commands: The decoded signals are converted into commands that can be used to control external devices or interfaces, such as a computer cursor, robotic prosthetics, virtual keyboards, or even synthesized speech.
Feedback: Sometimes the BCI provides feedback to the user, allowing him to see the results of his neural commands and adjust them if necessary.
Currently already widely used non-invasive types of BCI (read brain activity without surgery), these include:
Electroencephalography (EEG) – measures the electrical activity of the brain through electrodes attached to the scalp.
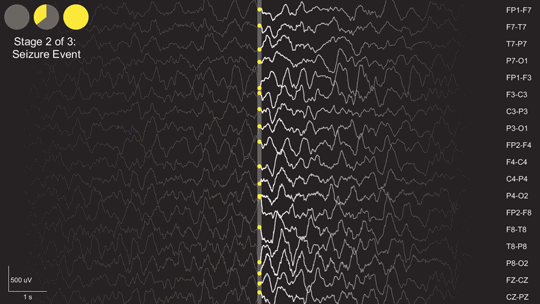
Functional magnetic resonance imaging – detects changes in brain blood flow associated with neural activity using a magnetic field and radio waves.
Magnetoencephalography — registers magnetic fields created by the electrical activity of the brain.
Functional near-infrared spectroscopy – measures blood flow in the cerebral cortex using infrared radiation.
Photoplethysmography (PPG) and other methods based on optical signals.
These technologies can be used to control computers, prosthetics, wheelchairs or other devices using brain signals. Non-invasive BCIs are considered more affordable and user-friendly, but they may be less accurate and have lower resolution compared to invasive methods such as implantable electrodes.
In a new study, scientists showed that an ALS patient participating in a clinical trial of an implantable brain-computer interface was able to speak understandable words that sounded like his own voice. Using real-time decoding of brain signals, the researchers synthesized speech based on activity in cortical areas associated with articulation and phonation. They focused on a limited vocabulary of six key words, which allowed them to achieve a high degree of intelligibility in their pronunciation.
Training of the decoding system lasted six weeks, after which the BCI was successfully used in several sessions.
The researchers also decided to provide delayed feedback, which avoids confusion or interference that may occur if the patient hears both their own voice and the synthesized voice from the BCI at the same time. This technology is ideal for maintaining communication even with progressive speech impairment due to ALS.
This breakthrough supports the feasibility of using BCI to restore speech in people with neurological disorders, starting with a limited number of words that the patient can reliably produce and expanding vocabulary in the future.
Main research approach
The study used technology to convert brain signals into acoustic speech.ri recurrent neural networks (RNN). The first RNN identified speech-related brain activity and buffered it. The second converted this activity into an intermediate acoustic representation. The third reconstructed the acoustic wave using a vocoder.
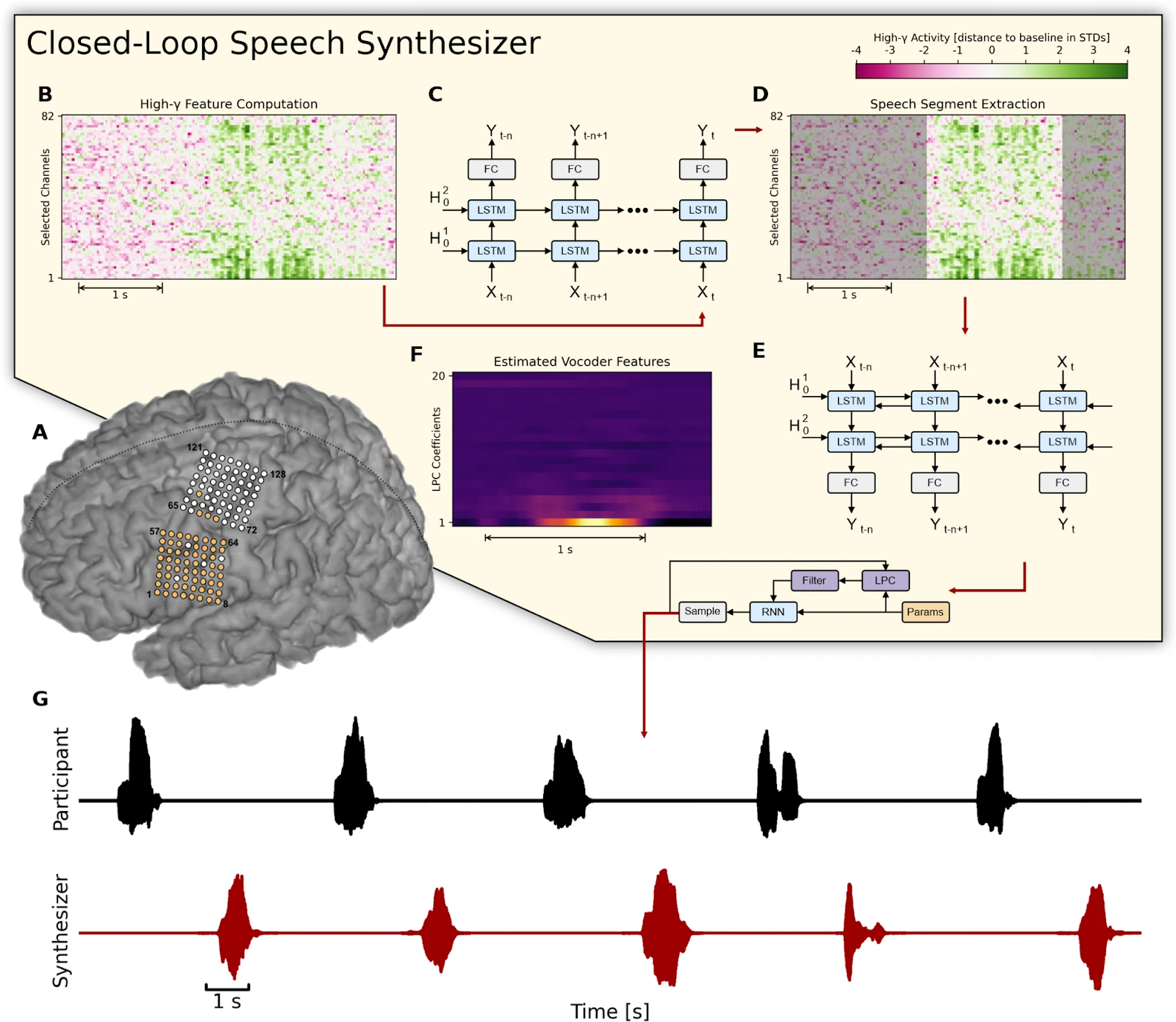
Electrocorticographic signals were obtained using special electrode arrays installed on the surface of the brain in areas responsible for speech production. (Fig. 1A)
Electrocorticographic signals are electrical signals that are recorded directly from the surface of the cerebral cortex. ECoG signals reflect the activity of neurons in the cerebral cortex and are usually obtained using a network of thin electrodes that are temporarily or permanently implanted during neurosurgery.
The researchers focused only on those electrodes that had previously been associated with increased activity in the high-frequency gamma wave range during word pronunciation.
The system analyzed the signals to identify patterns of brain activity associated with speech. These characteristics were then decoded into digital parameters, which were converted back into an acoustic speech signal using a vocoder. This synthesized speech signal was presented to the patient with a delay in the form of audio feedback.
This approach made it possible to create speech that was as close as possible to the patient’s natural speech, despite his neurological disease.
Research results
Synthesis system performance
In a study of a patient with ALS, the BCI speech synthesis system was able to accurately reproduce words similar to his natural speech. Figure 2A provides examples of original and synthesized sound waves, showing similarities in word onset and flow times. The analysis showed that the synthesis system corresponded to the timing of word pronunciation.
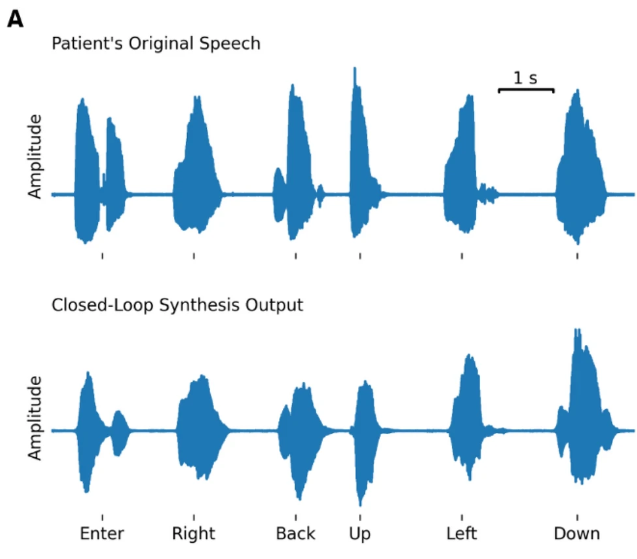
Figure 2B shows that the spectrograms of original and synthesized speech have many similarities, preserving phoneme and formant information.
Phoneme and formant information refers to key elements of the acoustic characteristics of speech that determine how we perceive different speech sounds.
Phonemes are the smallest units of sound in a language that can differentiate the meanings of words. For example, in English, the sounds /p/ and /b/ are different phonemes because they distinguish between the words “pat” and “bat”.
Formants are resonant frequencies in the vocal tract that enhance certain frequencies of sound produced by the vocal cords. They play an important role in the formation of vowel sounds. Each vowel has a characteristic set of formant frequencies that help us distinguish one vowel from another.
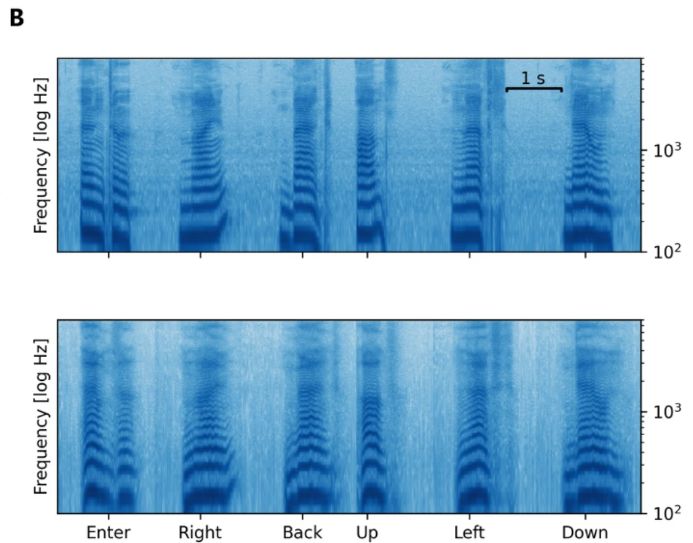
Tests conducted with listeners confirmed that most of the synthesized words were understandable and could be correctly recognized (achieving an accuracy rate of 80%).
The confusion matrix in Figure 2C showed high word recognition, with the exception of one word at a very high rate. The word “Back” was recognized at a low frequency, although above chance, and was most often mistaken for the word “Left.” This could be partly due to the close proximity of vowel formant frequencies in these two words.
*Please note that all keywords in the dictionary were chosen for intuitive control of a computer interface, such as a communication board, and were not designed to be easily distinguishable for BCI applications.
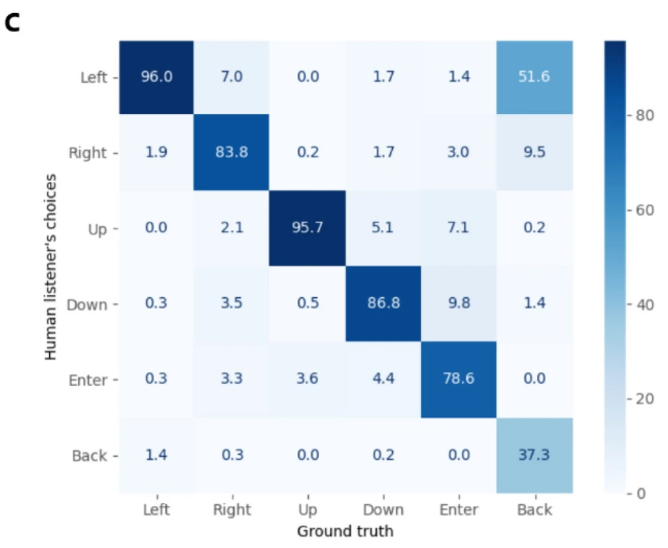
Figure 2D shows listeners' individual accuracy scores, with each listener recognizing more than 75% of the words.
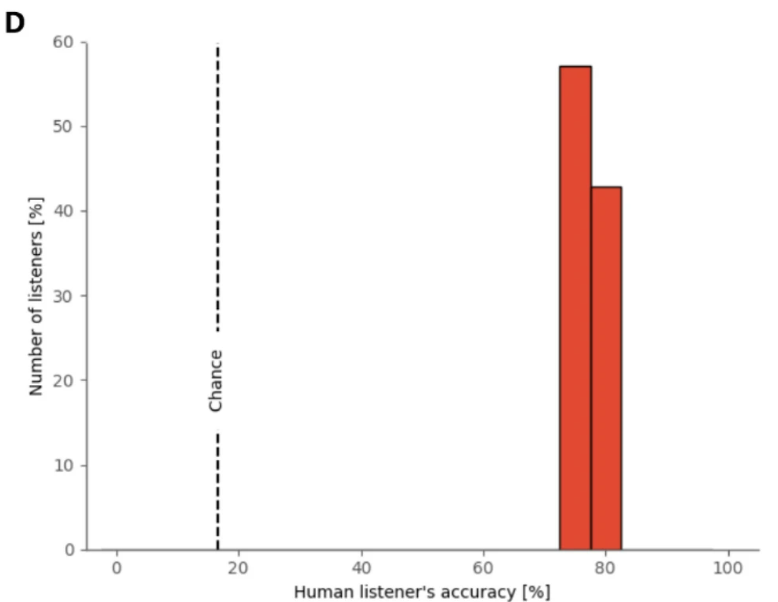
Next, the scientists conducted a significance analysis to understand which areas of the brain influence the recognition of speech segments. They used techniques from the field of image processing to determine which “pixels” contribute to speech classification, in this case which areas of the brain are active during speech. These data helped to evaluate the influence of high-gamma brain activity (frequencies 70–170 Hz) on speech onset (PSO) moments.
Figure 3B shows the process for assessing this impact. First, the moment of speech onset was determined, after which gradients were calculated indicating the degree of influence of each electrode.
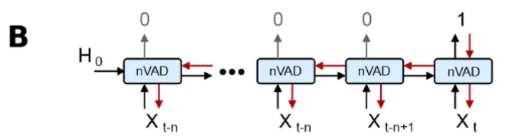
The results are presented in Figure 3A, where the size of the circle reflects the strength of the electrode's influence, and the color indicates the time of its maximum influence.
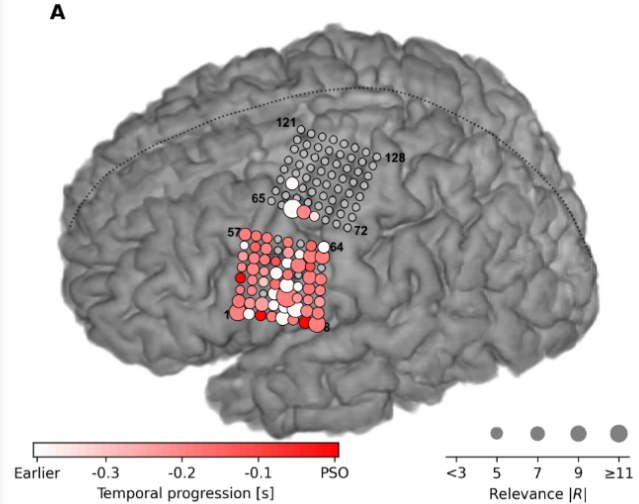
Significance analysis showed that the current nVAD model of neural detection of vocal activity used data from a wide network of electrodes located in motor, premotor And somatosensory areas of the brainfor speech detection.

Figure 3C shows results for three electrodes within one second before PSO (speech onset) that were strongly influential in predicting speech onset. These data, combined with the color coding from Figure 3A, indicate that the nVAD model effectively exploited neural activity during speech planning and phonological processing.
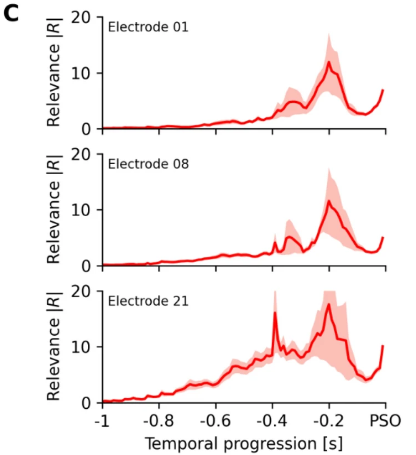
conclusions
I believe that scientists have been able to demonstrate the potential of using BCI in medicine: Clearly, BCIs can greatly help people with mobility impairments, such as those with ALS, stroke, or spinal cord injuries, by restoring their ability to communicate and interact with the world around them.
But, also, the use of BCI is acceptable in other areas, for example:
Prosthetics: BCIs are used to control robotic prosthetics, allowing users to control artificial limbs with their thoughts, allowing for more natural and intuitive control.
Rehabilitation: BCIs are used to rehabilitate stroke patients by stimulating brain plasticity and helping restore function by teaching the brain new ways to control movement.
Gaming industry and virtual reality: BCIs can improve interaction with video games and virtual environments by allowing users to control game characters or virtual objects directly with their thoughts.
Education and training: BCIs can be used to study learning and attention processes and to develop personalized learning programs.
Neuromarketing: Research using BCI provides an opportunity to better understand consumer preferences and responses to advertising messages by analyzing brain activity in response to marketing stimuli.
Condition and stress control: BCIs can help monitor mental state, for example to detect levels of stress or fatigue, which can be useful in the automotive industry or for professionals working in high-stress environments.
Thus, BCI has significant prospects in improving the quality of life of patients with various diagnoses, as well as increasing the efficiency of professional activities and opening new directions in technological progress.
You can view the main study at the following link: https://www.nature.com/articles/s41598-024-60277-2
That's all!
Thanks for reading, we'll be waiting for you in the comments 🙂