How AI can help diagnose skin cancer at an early stage
In recent decades skin cancer It is considered one of the most common cancers worldwide. According to WHO estimates, approximately 132,000 cases of skin melanoma, A mortality reaches 66,000 cases.
Diagnosing skin cancer requires significant effort and time, which can negatively affect the course of the disease and lead to disastrous consequences.
Fortunately, science does not stand still! In this article I will talk about system for early automated detection of skin cancer, using forces AI.
Happy reading! 🙂
Introduction to the Study
Skin cancer is a condition in which skin cells begin to divide abnormally and uncontrollably, which can lead to the formation of tumors. It can develop in any part of the skin, but most often occurs in areas exposed to ultraviolet radiation.
There are several types of skin cancer, including basal cell carcinoma, squamous cell carcinoma And melanoma. Basal cell carcinoma arises from the basal cells found in the lower layer of the epidermis and is often associated with prolonged sun exposure. Squamous cell carcinoma develops from cells in the top layer of skin and can be more aggressive and spread to other organs.
Melanoma is the most dangerous and deadly type of skin cancer that originates from melanocytes, the cells responsible for producing the pigment melanin. Melanoma has a high tendency to metastasize and rapidly spread cancer cells throughout the body.

There are many methods for diagnosing skin cancer. Traditional methods such as biopsy and regular medical checkup, have disadvantages: biopsy is an invasive and painful method that can lead to the spread of the disease; examination involves the physician's subjective judgment and may lead to errors in diagnosis. The accuracy of tumor discrimination is a limitation of traditional methods, especially in the early stages.
Access to dermatologists is also limited in many regions. In addition, the process of clarifying the diagnosis often requires significant time and can be expensive. Therefore, new technologies such as dermatoscopy, teledermatology And computer diagnostic support systems.
Usage machine learning can significantly improve the diagnostic process and help reduce processing time dermoscopic images.
The purpose of this work is to use automated algorithms based on AI for diagnosing skin cancer and determining the nature of neoplasms. Offered GUI (Graphical User Interface)which will simplify diagnosis for patients and doctors, reduce time for diagnosis and increase its accuracy!
Research methods
The paper presents an intelligent skin cancer detection system capable of classifying benign and malignant neoplasms.
Using the PH2 dataset with dermoscopic images allows the system to analyze different types of skin lesions.
The system includes stages of image preprocessing, segmentation, feature extraction and classification using machine learning algorithms implemented in MATLAB with a graphical interface for ease of use. The study results confirm that the automated approach can improve the diagnostic process and become an important tool in the early detection of skin cancer.

Preliminary processing
Image pre-processing for skin cancer diagnosis removes noise, improves contrast, and converts images to grayscale and filters them to improve quality. These steps help make the tumors more visible for later analysis.
Segmentation
Segmentation is a technique that can separate objects from their background, which can also accommodate the complex morphology of biological structures. At the segmentation stage, adaptive algorithms are used and modified. Adaptive snake (AS) And Region growing (RG).
Adaptive snake (AS) is an active contour model used to detect the edges and boundaries of objects in an image. It works by minimizing the energy function, which results in a “serpentine” curve that wraps around the object. This method is especially effective for selecting objects with complex contours.
Region growing (RG) is the process by which image pixels are grouped together based on predefined criteria such as intensity or color. This method is well suited for highlighting adjacent regions that have the same or similar characteristics.
Feature Extraction
To analyze the affected skin areas, a feature extraction method based on gray level spatial dependence matrix (GLCM)which characterizes the texture of the image.

This method provides statistical information about texture, including parameters such as contrast, uniformity and entropy.
Also at this stage, the ABCDE rules of dermatology are applied, evaluating the asymmetry, borders, color, diameter and shape of skin lesions, which is important for detecting early signs of cancer.
Classification
At this stage, algorithms are used ANN And SVM for classification of skin lesions.
ANNs (Artificial Neural Networks) are computing systems inspired by biological neural networks, consisting of a large number of simple, interconnected nodes working together to solve specific problems. ANNs are able to learn and improve their performance based on input data through a process that mimics the way the human brain processes information.
SVM (Support Vector Machines) is a set of related supervised learning methods used for classification and regression analysis. The basic idea of SVM is to find a hyperplane in a multidimensional space that best separates the data into different classes. SVM deals effectively with high-dimensional data and provides high accuracy by using various kernel functions that can solve nonlinear problems.
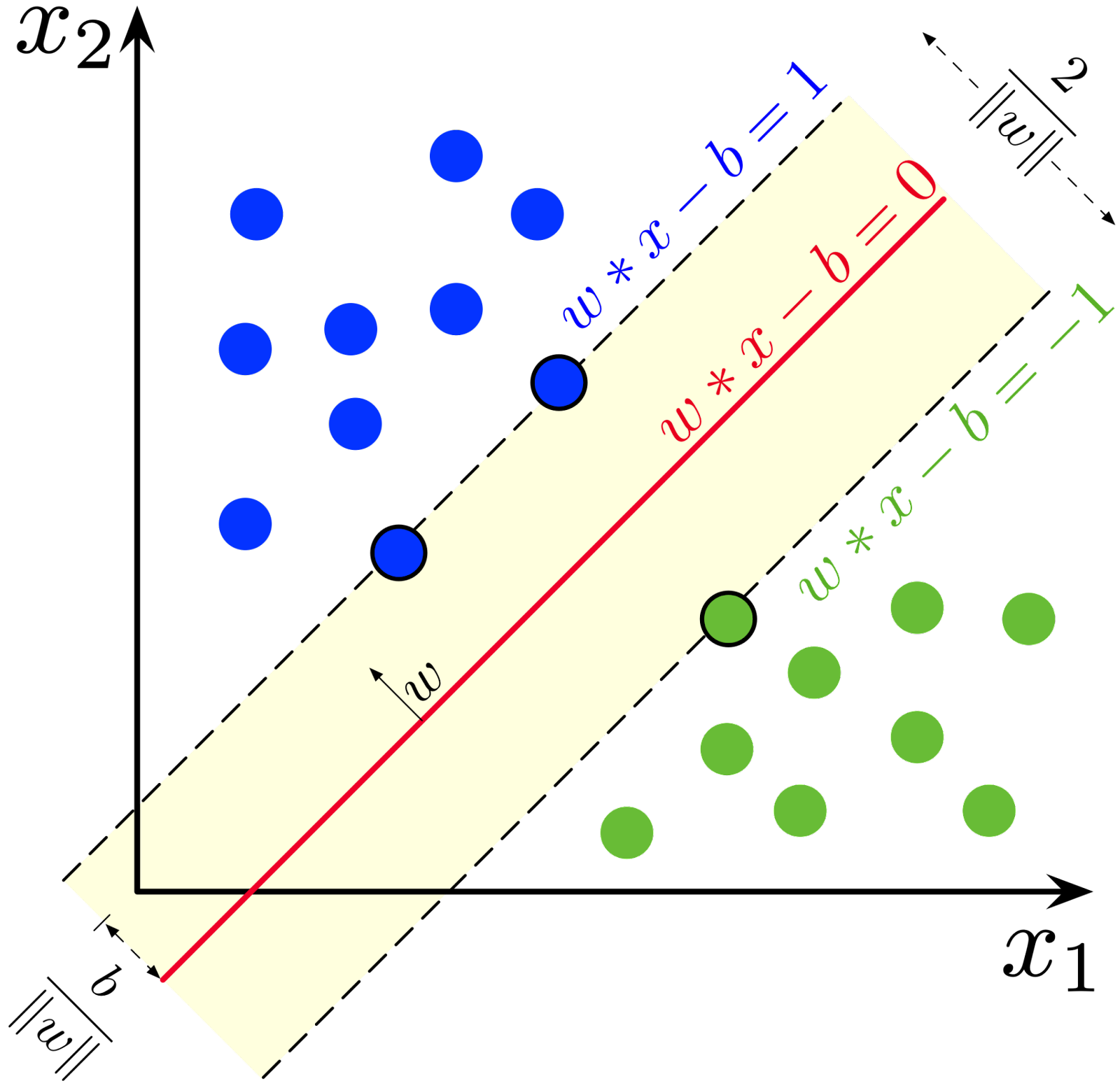
In the classification process for skin cancer detection, ANN and SVM algorithms are trained using labeled datasets containing various features extracted from images of skin lesions, such as color, texture, and shape information. Once trained, these algorithms can classify new images of skin lesions into different categories, such as benign or malignant, based on learned patterns and relationships in the data.
Grade
Evaluation metrics play an important role in the development, validation, and implementation of skin cancer detection algorithms. They provide key information about algorithm performance, support clinical decision making, and ultimately improve patient outcomes.
The effectiveness of the proposed system is assessed using the following metrics:
Accuracy;
Sensitivity;
Specificity;
F1 indicator;
Jaccard index;
Matthews correlation coefficient.
Research results
In this figure, the algorithm is used for segmentation AS. Its accuracy is 96%!
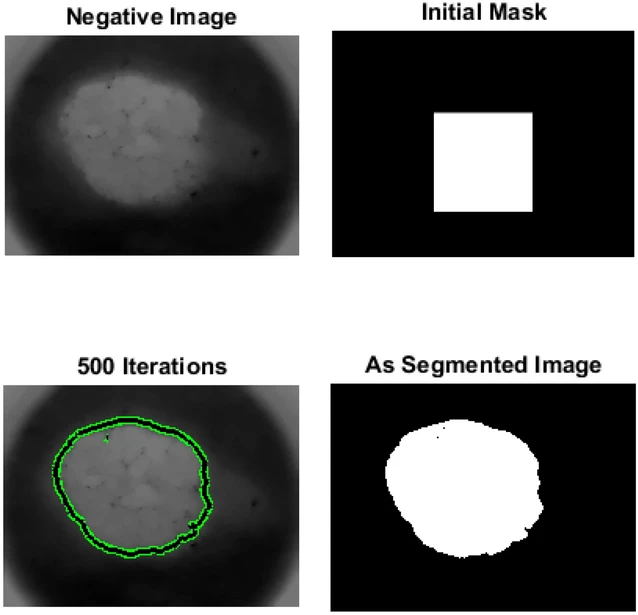
Segmentation accuracy using RG reaches 90%.

AS shows better effectiveness due to its flexibility, ability to incorporate previous knowledge, and ability to deal with complex lesions.
AS provides more accurate boundaries compared to RG, especially for objects with irregular boundaries or complex shapes. AS is more suitable for a wider range of images.
RG can segment an image if it is symmetrical, but is slower than AS and cannot segment an image if it is asymmetrical.
The figure below shows the graphical interface for the proposed system:

The proposed system with the ANN algorithm has the maximum efficiency (accuracy – 94%; specificity – 95.83%; sensitivity – 92.30%; F1 score – 0.94) compared to SVM.
The proposed system is easy to use, saves time, allows patients to independently monitor remotely, and has high performance. This system speeds up the work of dermatologists and increases the efficiency of diagnosis.
Conclusion
Of course, this system still requires improvements, but already at this stage it is becoming an effective method for early diagnosis of skin cancer.
It is important to clarify that it cannot replace doctors, and cannot be the only unconditional source of diagnosis. This is a great tool that can really make life a lot easier for both professionals and patients. The use of this method can provide diagnosis of tumors at early stages, which will simplify treatment and rehabilitation and, accordingly, reduce mortality.
You can view the main study at the following link: https://www.nature.com/articles/s41598-024-59783-0#Tab1
That's all!
Thanks for reading, we'll be waiting for you in the comments! 🙂