Can ChatGPT replace a doctor's visit? We check the effectiveness of ChatGPT in determining the diagnosis and choosing treatment
Nowadays people solve many important problems using forces. artificial intelligencehowever, health issues are always acute and require more qualifications.
Is it possible to replace a visit to the doctor with a visit to ChatGPT? Surely many people have had a disastrous experience using Google, when, in response to simple symptoms of food poisoning, you were provided with all sorts of types of cancer as a probable diagnosis.
Is there a chance to avoid this problem and get correct recommendations on the prospects for examinations and treatment options (with the formulation of several of the most realistic diagnoses!)?
In this article I will tell about ChatGPT testing as a qualified specialist in making the correct diagnosis, determining the list of necessary examinations and treatment paths. All research methods and its results will be presented in detail below!
Happy reading 🙂
Introduction to the Study
Language models, such as ChatGPT, were not originally developed for medical purposes, but research points to their potential in this area. ChatGPT was able to successfully pass the USMLE exam required to obtain a medical license in the United States. This paves the way for the use of AI to support physician activities and patient care.
In the new study, researchers focused on assessing the accuracy GPT-3.5 and GPT-4, in three main tasks: making a primary diagnosis, providing recommendations on necessary examinations and choosing treatment. Particular attention is paid to rare diseases, which, although infrequent, collectively affect a large proportion of the population and require diagnostic support.
The study uses clinical descriptions tailored for lay audiences and derived from licensed sources, reducing the likelihood that ChatGPT was pre-trained on this data.
Researchers also compared the capabilities of AI with a search engine Google and evaluated open models such as Llama 2noting their superiority in general chat tasks.
Overall, the study aims to comprehensively evaluate the capabilities of the latest language models in facilitating the diagnosis and treatment of diseases of varying degrees of prevalence, which has the potential to revolutionize medical practice and provide doctors with a powerful tool to improve the quality and accessibility of healthcare. In addition to improving diagnosis, AI can help doctors interpret complex medical images, manage patient data, and provide personalized treatment recommendations.
Research methods
Selection of case reports
To conduct the study, case histories published in German collections from two publishers: Thieme and Elsevier were studied. The main clinical areas were surgery, neurology, gynecology and pediatrics. Two more casebooks from Elsevier, focusing on rare diseases and general medicine, have also been added to further include cases of very low incidence and outpatient conditions. As a result of selection 1020 cases were selected.
To study the performance of ChatGPT depending on the frequency of diseases, cases were divided into three subgroups: the disease was considered frequent or less frequent, if its frequency exceeded 1:1000 or 1:10,000 per year, respectively. A disease was considered rare if the incidence was below 1:10,000. If frequency information was not available, the disease was considered rare. Power calculations were performed to determine a sample size of 33–38 cases per subgroup to achieve an overall power of 0.9.
To limit the scope of subsequent analysis, a random sample of 40% of the total number of 1020 cases was conducted to ensure an even distribution of sources, resulting in 408 cases were studied.
The selection of cases for further analysis was carried out by specialists. Only those cases that met the following requirements were included: (1) the patient or someone else can provide medical history information (eg, excluding patients with severe trauma), (2) no photos required for diagnostic purposes, (3) diagnosis does not rely too much on laboratory tests(4) the case is not a duplicate.
Steps 2 and 3 were necessary because the diagnostic task was only to evaluate the initial diagnosis, in which the patient does not have any imaging or laboratory findings. A total of 153 cases meeting the established inclusion criteria were identified.
To ensure balanced representation, the researchers sought to include an equal number of cases from each medical specialty, taking into account both incidence rates and publication source, resulting in a final set of 110 cases was made.
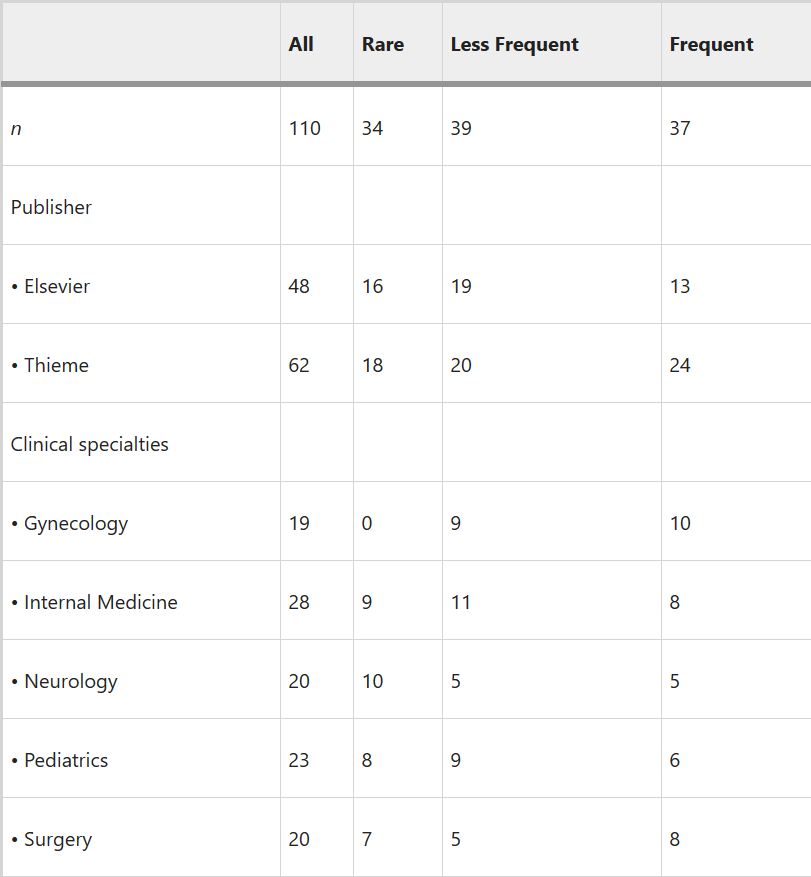
In order to simulate the actual patient situation, cases were processed in lay language. The case history was presented in the first person, contained only general information, and avoided the terminology of clinical experts.
Request GPT-3.5 and GPT-4
To generate patient requests, the analysis plan is as follows:
Open a new ChatGPT dialog;
Probable Diagnosis: Record the patient's medical history and current symptoms, add “What are the most likely diagnoses? Name up to five pieces”;
Screening Options: “What are the most important tests to consider in my case? Name up to five pieces”;
Open a new ChatGPT dialog;
Treatment Options: Write down the patient's medical history and current symptoms and add: “My doctor diagnosed me with (specific diagnosis X). Which treatment methods are most suitable for my case? Name up to five.”
Google Query
A search for symptoms was conducted and the most likely diagnosis was determined based on the first 10 results reported by Google. Search, retrieval, and interpretation were performed by a non-medical professional simulating the patient's situation.
Only information available on websites was assessed. Further detailed searches for a specific diagnosis were not performed if, for example, the website provided only limited information about disease characteristics.
Further research using Llama 2
The scientists used Llama 2 with two different model sizes: Llama-2-7b-chat (Ll2-7B with 7 billion parameters) and Llama-2-70b-chat (Ll2-70B with 70 billion parameters). Patient queries were generated similarly to GPT-3.5 and GPT-4, starting with the system prompt “You are a helpful assistant,” followed by a query format consistent with that used for GPT.
All queries were formulated with certain parameters: temperature set to 0.6, top_p set to 0.9, and maximum sequence length 2048.
Efficiency mark
The responses obtained using GPT-3.5, GPT-4, Google, Ll2-7B and Ll2-70B were assessed by two independent clinicians. Each physician rated clinical accuracy on a 5-point Likert scale according to the table. The final score is calculated as the average of the two individual scores.

To determine interrater reliability, weighted Cohen's kappa coefficients and 95% confidence intervals were calculated for each of the three tasks using the R package DescTools 0.99.54.
An independent evaluation of diagnostics, testing options, and treatment options was conducted to examine the performance of GPT-3.5, GPT-4, and Google. The performance of GPT-3.5 compared to GPT-4 was evaluated using one-sided Mann-Whitney test. To make a diagnosis, paired one-sided Mann-Whitney tests were additionally performed comparing GPT-3.5 with Google and GPT-4 with Google. Possible frequency effects were examined using an unpaired one-tailed Mann-Whitney test.
The Mann-Whitney test, also known as the Wilcoxon test, is a nonparametric statistical testing method that is used to compare two independent samples. It is designed to determine whether there are significant differences between two groups with respect to their central tendency (e.g., median).
Research results
Inter-rater reliability
*Inter-rater reliability is a statistical measure used to determine the degree of agreement between different raters. It is necessary to determine how consistent different versions of AI language models are when making a diagnosis, recommending examinations and choosing treatment.
As a measure of inter-rater reliability, the researchers used Cohen's Kappa coefficient (k). It takes into account the possibility of agreement by chance, and is therefore considered more reliable than simple percentage agreement. In addition, the coefficient can vary from 0 (complete disagreement) to 1 (complete agreement). In accordance with the developed classification, different values are assessed as:
0.81–1.00—almost perfect agreement;
0.61–0.80—substantial agreement;
0.41–0.60—moderate agreement;
0.21–0.40—fair agreement;
0.00–0.20—weak agreement;
<0.00—no agreement.
Regarding diagnosis, the highest levels of agreement are observed when k=0.8 for GPT-3.5, k=0.76 for GPT-4 and k=0.84 for Google. Competent recommendations for inspection are characterized by values k=0.53 for GPT-3.5 and 0.64 for GPT-4. In relation to treatment there is k=0.67 for GPT-3.5 and k=0.73 for GPT-4.
According to the classification, the results correspond to a level of agreement from significant to almost ideal. The best indicators relate to the possibility of making a diagnosis.

Performance evaluation of GPT-3.5, GPT-4 and Google
The figure below summarizes the pairwise comparisons and also shows performance for each of the three disease incidence subgroups (cumulative frequency graphs).
Cumulative frequency graphs are visual tools for representing the accumulated (cumulative) number of cases or observations that meet a certain criterion or fall into a certain category. These graphs show how data accumulates as you move along one of the axes.
These graphs typically display the cumulative number of cases on the Y-axis and the values over which the accumulation occurs, such as accuracy scores, on the X-axis. Using different shades of color in a graph can help visually separate data into subgroups, for example, by disease incidence:
Light blue represents rare diseases;
Medium blue color – less frequent;
Dark blue color – frequent diseases.

The performance distribution of all models for various tasks is summarized using bar charts and violin plots:


Regarding diagnosis, all three instruments were assessed. Pairwise comparison showed significantly better performance of GPT-4 (median: 4.5, IQR = [3,81; 4,75]) vs. as GPT-3.5 (median: 4.25, IQR = [3,0; 4,75]p = 0.0033) and Google (median: 4.0, IQR = [2,75; 4,75], p = 0.0006). However, no significant difference was observed between GPT-3.5 and Google.

Considering disease frequency, the plots in Figure 4a show consistently better performance for common versus rare diseases. This observation was made for all instruments (the dark blue line – frequent – rises steeper compared to the light blue line – rare). GPT-3.5 performed significantly better on common compared to rare diseases (p < 0.0001), while GPT-4 showed significant results for both common and rare (p = 0.0003), as well as less frequent and rare (p = 0.0067). For Google, no differences were observed between rare and less common diseases.
Considering the potential of screening recommendations, GPT-4 was compared (median: 4.5, IQR = [4,0; 4,75]) and GPT-3.5 (median: 4.25, IQR = [3,75; 4,5]). Pairwise comparison showed superior performance of GPT-4 (p < 0.0001). Evaluating the performance of the two models on disease incidence, the results showed the effectiveness of GPT-3.5 on frequent disease. However, these results were not significant. GPT-4 showed comparable performance in both common and less frequent diseases, but significantly better performance compared to rare diseases (p = 0.0203).
Regarding the ability to provide treatment options, the effectiveness of GPT-4 was compared (median, 4.5, IQR = [4,0; 4,75]) and GPT-3.5 (median: 4.25 (IQR = [4,0; 4,69]). In this situation, fewer differences were observed. The results in Figure 4c showed better, but not significant, performance of GPT-4 (p = 0.0503). No effect of disease frequency on performance was observed.
Comparison with Llama 2
The figure below visualizes the performance of GPT-3.5 and GPT-4 with violin plots considering all 110 cases and dots highlighting the performance of 18 selected cases compared to Llama-2-7b-chat (Ll2-7B) and Llama-2-70b -chat (Ll2-70B).

The performance of all models divided by disease frequency is shown below.

The median estimates and interquartile ranges for the top nine cases were 14.5 [14,5; 14,75]14.0 [13,25; 14,0]12.25 [11,25; 13,5] and 11.75 [11,25; 12,75] for GPT-4, GPT-3.5, Ll2-7B and Ll2-70B, respectively. Likewise, for the nine worst cases the scores were: 11.0 [9,25; 11,25]10.25 [9,5; 10,5]10.25 [8,5; 11,0] and 8.5 [7,75; 10,25].
Overall, one can observe slightly worse performance of open language models compared to GPT-3.5 and GPT-4. Additionally, there is no noticeable difference in performance between the two open language model configurations.
Conclusion
This study demonstrated that artificial intelligence models such as GPT-3.5 and GPT-4 show significant improvements in performance compared to naive Google search in clinical decision support. However, even with high median scores, these models may not always achieve positive results in complex tasks, such as initial diagnosis.
It is also important to note that using Llama 2 may require fine tuning and updates to maintain high performance in medical scenarios. Such models, despite small performance improvements, may be more inconsistent than commercial counterparts such as ChatGPT.
The authors of the main study are Sarah Sandmann And Sarah Riepenhausen: https://www.nature.com/articles/s41467-024-46411-8
You can also read my previous article about Possibility of using GPT-4 for RNA sequencing: https://habr.com/ru/companies/bothub/articles/805869/
That's all!
Thanks for reading! We will be waiting for you in the comments 🙂